Comprehensive Guide to UA Data Backup
Backup of Data
As of July 1, 2024, the storage of Universal Analytics (GA3) data will cease, representing a pivotal moment in data management practices. It’s crucial for organisations to comprehend the importance of preserving this data for future analysis and decision-making processes. Historical trends within UA data offer invaluable insights into user behaviours, preferences, and patterns over time.
Therefore, it’s essential for businesses to proactively store or download UA data before the cutoff date and continue leveraging it for informed decision-making and strategic planning endeavours.
Steps to backfill the data
Here’s a step-by-step guide to backfill the UA data to ensure no data is lost:
-
Identify Data to Backup
The initial and most crucial step involves determining which data to preserve, especially given the significant volume of data within UA. To streamline this process, we’ve categorised the data into two distinct parts, facilitating the development of your personalised blueprint.
-
Primary level data:
The primary data encompasses information of utmost importance for the organization. Therefore, it’s imperative to securely store this data. This includes:
– Conversion events, alongside metrics such as Total Events and Total Users.
– Traffic-related insights detailing various channels through which users access the site. This can be further segmented into specific source/medium categories.
– Campaigns that attract users, including metrics like total users, total events, and conversions.
– Key funnels such as the purchase funnel, checkout funnel, and other journey-related funnels. Analysing these helps in understanding historical trends over time.
– Key Performance Indicators (KPIs) essential for the organization, comprising custom dimensions and metrics developed over time to gather organization-specific data.
-
Secondary level data:
Secondary level data comprises important information, though it may not be immediately necessary unless the site relies heavily on content. This includes:
– Analysis of most-read blogs/newsletters on the site, including metrics such as total users, average session duration, sessions, and bounce rate.
– Assessment of average page load time to gauge site performance, alongside pageviews.
– Demographic data such as age and gender, providing insights into user-specific demographics for further evaluation.
-
Evaluate the time frame
This step is crucial as it determines the volume of data to be stored. A shorter time frame results in less data and consequently requires less storage space, while a longer timeframe necessitates more storage space.
Typically, a two-year data retention period is considered adequate for analyzing historical trends and assessing significance. This timeframe is widely accepted by most organizations.
-
Select the method for data backfilling
Select the method for data backfilling wisely to ensure comprehensive data recovery and continuity in analysis.
1. Manually export data
Google Analytics allows you to download the data you need for further work using an EXPORT button above the date range in each report.
Open the Google Analytics report you want to save. For example, Acquisition > All Traffic > Source/Medium. You can then apply additional settings: add a segment, a filter, or another parameter for the report.
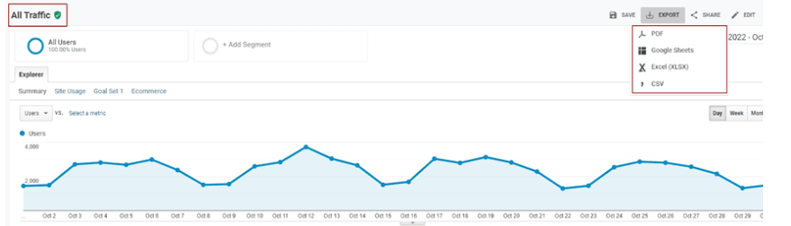
UA backfilling
Next, in the upper right corner, click the EXPORT button. Select the file format from the drop-down menu: PDF, Google Sheets, Excel (XLSX), or CSV.
Limitation
- A maximum of 5,000 rows can be downloaded, aligning with the display limit in the Google Analytics interface. Additional data will be aggregated as “(other)”, necessitating alternative export methods.
- High daily visit volumes may result in data sampling, impacting the accuracy of exported data.
2. Export data using the Google Analytics Spreadsheet Add-on (API)
For Universal Analytics (UA), Google offers its own Google Analytics add-on, enabling access to data directly within Google Sheets.
With the Google Analytics Spreadsheet Add-on, you can:
– Retrieve data from multiple views
– Perform custom calculations based on report data
– Generate visualizations using built-in tools and embed them on third-party websites
This serves as a viable alternative, especially when data backfilling is specified. However, note that creating a report may take some time, particularly when narrowing down the data.
Limitations
The limitations of exporting data using the Google Analytics Spreadsheet Add-on (API) include:
1. Data Volume: The add-on may have limitations on the amount of data that can be exported in a single request. Large datasets may need to be split into multiple requests, which can increase processing time and complexity.
2. API Quotas: Google Analytics API has usage quotas, including limits on the number of requests per day and per user. Exceeding these quotas may result in data export failures or temporary restrictions on API access.
3. Sampling: When exporting large datasets, Google Analytics may apply data sampling to speed up processing.
3. Exporting the Google Analytics data through Python
To extract Universal Analytics (UA) data via API and export it to a CSV file using Python, certain prerequisites need to be met:
– Visual Studio Code is essential for coding and script manipulation.
– Proficiency in Python programming is a prerequisite.
Note:
When retrieving data for extended periods, such as 3-4 years, a higher sampling rate is applied, though it’s lower than that in the GA Portal. To ensure greater data accuracy, it’s advisable to fetch the data quarterly to mitigate excessive sampling.
Limitations
When backfilling User Acquisition (UA) data via Python, there are several limitations to consider:
- API Quotas: If you’re using APIs to fetch UA data, there are often limits on the number of requests you can make within a given time period.
- Rate Limits: Similar to API quotas, there may be rate limits imposed by the data source or API provider. Exceeding these limits can result in throttling or temporary bans on API access.
- Data Processing Time: Processing time can vary depending on factors such as the size of the dataset, the complexity of the queries, and the performance of the underlying infrastructure.
4. Exporting the Google Analytics data to the Big Query
Exporting Universal Analytics data to BigQuery requires the following prerequisites:
– A Google Cloud Platform account with billing enabled.
– A BigQuery project with billing enabled to store the UA data.
– Access to Supermetrics, a third-party connector solution facilitating the transfer of UA data to BigQuery, as the API is restricted to UA 360 users and not available to the general user base.
– Proficiency in SQL is necessary to execute queries for UA data within BigQuery.
Limitations
- Data Transfer Costs: Exporting UA data to BigQuery can incur data transfer costs, especially if you’re dealing with large datasets or frequent data updates.
- Data Volume and Storage: Large datasets may require additional storage capacity and processing resources, which can increase costs and impact performance.
-
Choose Storage Option
Once you’ve chosen the method, the next step is determining the storage location for the data, ensuring convenient visualization and analysis whenever required.
a. Cloud Storage Solutions:
– Google Cloud Storage offers a free tier with limited storage and access to various features. Users can store UA data securely and scale storage as needed.
– Amazon S3 also provides a free tier with limited storage and access to beginners. It’s a reliable option for storing backups of UA data with options for data retrieval and management.
b. On-Premises Storage Systems:
– Utilizing existing on-premises storage infrastructure incurs no additional cost apart from maintenance and operational expenses. This option provides full control over data storage and security, ideal for organizations with stringent compliance requirements.
c. Cloud Data Warehouses:
– Cloud data warehouses like Google BigQuery, Amazon Redshift, or Snowflake offer scalable and high-performance storage solutions for the analytical processing of UA data.
In summary, the backup of data underscores the critical importance of preserving valuable information for future use. Choosing the right method ensures data integrity and accessibility, enabling organizations to navigate challenges, make informed decisions, and drive success with confidence.